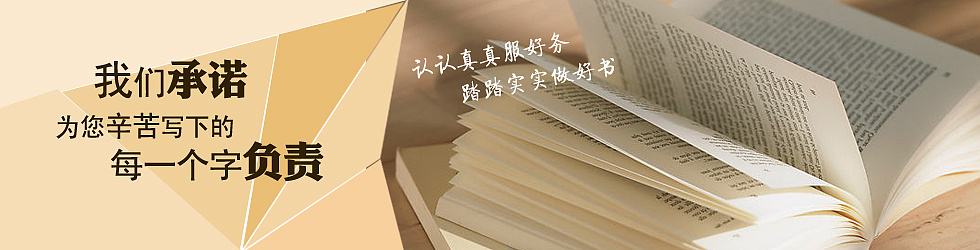
客服中心
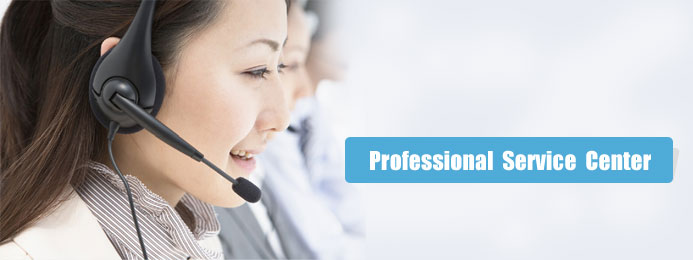
咨询电话:+65 62233778
24小时服务电话: +65 62233778
投诉监督:+65 62233778
投稿邮箱:2355654820@qq.com 3003381682@qq.com
最新期刊更新
A Logistic Regression Model to Predict Graduate Student Matriculation
来源:世界期刊网 时间:2023-02-01 16:50:58点击:247
Abstract
Higher education institutions invest a significant amount of resources every year to recruit new students. However, higher education administrators have been continuously facing challenges in enrollment management due to the demographic shifts, dramatic increases in educational costs, intense competition among institutions, and the uncertain nature of human selection patterns (Baum, Kurose, &McPherson, 2013).[3] Today's post-baccalaureate applicants are more knowledgeable than in previous years, because they can access information on a specific graduate program, in a given college, at any time. As reported in numerous studies, the number of graduate students switching out of their universities continues to be an essential issue. A useful prediction model of matriculation that uses available student data is highly desirable to assist the graduate students with timely advising early in their universities. This study was designed to build a predictive model for the probability that a specific admitted graduate student will matriculate. The results indicated that ten predictive variables were statistically significant at the .05 level. Getting an assistantship made the most substantial positive contribution in predicting student matriculation, followed by FAFSA, experience with the university, campus, degree level, college, gender, age, the number of days between application and admission, and distance to the university. This study's results could be beneficial for improving marketing efforts aimed toward individuals with characteristics most likely to enroll. Administrators could calculate the predictive score (or percentage) for each prospective student based on the predictive model. Marketing efforts could then concentrate on those applicants whose predictive score is high and eliminate the low qualifying students from their recruitment plan.
Keywords
Matriculation; Graduate Admission; Financial Aid; Enrollment
References
[1] Allum, J., & Okahana, H. (2015). Graduate enrollment and degrees: 2004 to 2014 [PDF]. Washington, DC: Council of Graduate Schools.
[2] Astin, A. W. (1984). Student involvement: A developmental theory for higher education. Journal of College Student Personnel, 25(4), 297-308.
[3] Baum, S., Kurose, C., Mcpherson, M. (2013). An overview of American higher education. The Future of Children, 23(1), 17-39.
[4] Bean, J. P. (1983). The application of a model of turnover in work organizations to the student attrition process. The Review of Higher Education, 6(2), 129-148.
[5] Bogard M., Helbig, T., Huff, G., & James, C. (2011). A comparison of empirical models for predicting student retention. Bowling Green: Western Kentucky University.
[6] Bohannon, T. (2007, April 16). Predictive Modeling in Higher Education. Speech presented at SAS Global Forum in Walt Disney World Swan and Dolphin Hotel, Orlando, Florida.
[7] Bruggink, T., & Gambhir, V. (1996). Statistical models for college admission and enrollment: A case study for a selective liberal arts college. Research in Higher Education, 37(2), 221-240.
[8] Burkhardt, J., DesJardins, S., Teener C., Gay, S., & Santen, S. (2016). Enrollment management in medical school admissions: A novel evidence-based approach at one institution. Academic Medicine 91(11) 1561-1567.
[9] Burtner, J. (2005). The use of discriminant analysis to investigate the influence of non‐cognitive factors on engineering school persistence. Journal of Engineering Education, 94(3), 335-338.
[10] Cabrera, A. F., Nora, A., & Castaneda, M. B. (1993). College persistence: Structural equations modeling test of an integrated model of student retention. The Journal of Higher Education, 64(2), 123-139.
[11] Defining Applicants as International or Domestic. (n.d.). Retrieved October 15, 2016, from http://www.grad.illinois.edu/admissions/instructions/02b
[12] Delisle, J., Phillips, O., & van der Linde, R. (March, 2014). The graduate student debt review: The state of graduate student borrowing [PDF]. New America Education Policy Program.
[13] DesJardins, S. (2002). An analytic strategy to assist institutional recruitment and marketing efforts. Research in Higher Education, 43(5): 531-553.
[14] Flaherty, C. (2015, September 17). Going Back to School. Inside Higher Ed. Retrieved September 21, 2016, from https://www.insidehighered.com/news/2015/09/17/study-finds-first-time-enrollment-graduate-school-35-percent
[15] French, B. F., Immekus, J. C., & Oakes, W. C. (2005). An examination of indicators of engineering students' success and persistence. Journal of Engineering Education, 94(4), 419-425.
[16] Garza, A.N., & Fullerton, A.S. (2018). Staying lose or going away: how distance to college impacts the educational attainment and academic performance of first-generation college students. Sociological Perspectives, 61(1), 164-185.
[17] Goenner, C., & Pauls, K. (2006). A predictive model of inquiry to enrollment. Research in Higher Education, 47(8), 935-956.
[18] Henley, G. (n.d.) Predicting successful matriculation through basic sciences [PDF]. Retrieved from http://www.gs.howard.edu/middle_states/mse/supportingdocs/chapter%2010%20-%20institutional%20assessment/10.7%20Case%20Study%20-%20Predicting%20Successful%20Matriculation%20through%20Basic%20Sciences.pdf
[19] Hosmer, D., & Lemeshow, S. (2000). Applied Logistic Regression (2nd edition). New York: Wiley.
[20] Jeffe, D. & Andriole, D. (2011). A national cohort study of MD-PhD graduates of medical schools with and without funding from the national institute of general medical sciences’ medical scientist training program. Careers in Academic Medicine 86(8), 953-961.
[21] Khajuria, S (2007). A model to predict student matriculation from admissions data (Master’s thesis). Retrieved from Ohio University database http://rave.ohiolink.edu/etdc/view?acc_num=ohiou1167852960
[22] Leppel, K. (1993). Logit estimation of a gravity model of the college enrollment decision. Research in Higher Education, 34 (3), 387-398.
[23] Leslie, L. & Brinkman, P. (1987). Student price response in higher education: The student demand studies. The Journal of Higher Education, 58, 181-204.
[24] Pallant, J. (2007). SPSS survival manual: A step by Step guide to data analysis using SPSS for windows (Third). New York: MCGraw-Hill Higher Education.
[25] Paulsen, M. B. (1990). College choice: Understanding student enrollment behavior. ASHE-ERIC Higher Education Report No.6. Washington, DC: The George Washington University, School of Education and Human Development.
[26] Pritchard, R., Klumpp, M., & Teichler, U. (Ed.). (2015). Diversity an excellent in higher education. Springer.
[27] Prytherch, D., Briggs, J., Weaver, P. C., Schmidt, P., & Smith, G. B. (2005). Measuring clinical performance using routinely collected clinical data. Medical Informatics and the Internet in Medicine, 30(2), 151-156.
[28] Sampath, V., Flagel, A., & Figueroa, C. (2009, October). A logistic regression model to predict freshmen enrollments. Paper presented at the annual meeting of the SouthEast SAS Users Group. Retrieved from http://analytics.ncsu.edu/sesug/2009/SD016.Sampath.pdf [Google Scholar]
[29] Singell, D. (2004). Come and stay a while: does financial aid effect retention conditioned on enrollment at a large public university? Economics of Education Review, 23(5), 459-471.
[30] Shanghai Ranking Consultancy (2009). Academic rankings of world universities. Shanghai, China: Shanghai Jiao Tong University. Retrieved from http://www.arwu.org/
[31] Snyder, T.D., de Brey, C., & Dillow, S.A. (2018). Digest of Education Statistics 2016 (NCES 2017-094). National Center for Education Statistics, Institute of Education Sciences, U.S. Department of Education. Washington, DC.
[32] St. John, E., Kirshstein, R., and Noell, J. (1991). The impact of student financial aid on persistence: A sequential analysis. Review of Higher Education, 14(3): 383–406.
[33] St. John, E. (2000). The impact of student aid on recruitment and retention: What the research indicates. New Directions for Student Services, 89, 61-75.
[34] Terkla, D., & Jackson, G. (1984). Conceptual models of student choice. Boston: Harvard University.
[35] The Average Student Loan Debt (2015). Retrieved September 30, 2016, from http://www.collegescholarships.org/loans/average-debt.htm
[36] The Institute for College Access and Success. (2016). Student debt and the class of 2015. Retrieved from: https://ticas.org/wp-content/uploads/legacy-files/pub_files/classof2015.pdf
[37] Thomas, E., Dawes, W., & Reznik, G. (2001). Using predictive modeling to target student recruitment: Theory and practice. AIR Professional File 78(Winter): 1–8.
[38] Tinto, V. (1975). Dropout from higher education: A theoretical synthesis of recent research. Review of Educational Research, 45(1), 89-125.
[39] Veenstra, C. P., Dey, E. L., & Herrin, G. D. (2009). A Model for Freshman Engineering Retention. Advances in Engineering Education, 1(3), 1-33.
[40] Wendler, C., Bridgeman, B., Cline, F., Millett, C., Rock, J., Bell, N., & McAllister, P. (2010). The Path Forward: The Future of Graduate Education in the United States. Princeton, NJ: Educational Testing Service